We worked with the London Fire Brigade (LFB) and National Fire Chiefs Council to explore the impact of machine learning for predicting fires – by building an address catalogue with complex details of every building in London.
Working with the ASI Fellowship, the LFB used the UPRN as the common identifier, as they brought details together from several different sources – Ordnance Survey, LIDAR data, data from the ONS, Energy Performance Certificates and their own fire history data. This new, richer dataset enabled the team to use machine learning that would not only predict that fire risk, but also analyse the impact of having the UPRN in place.
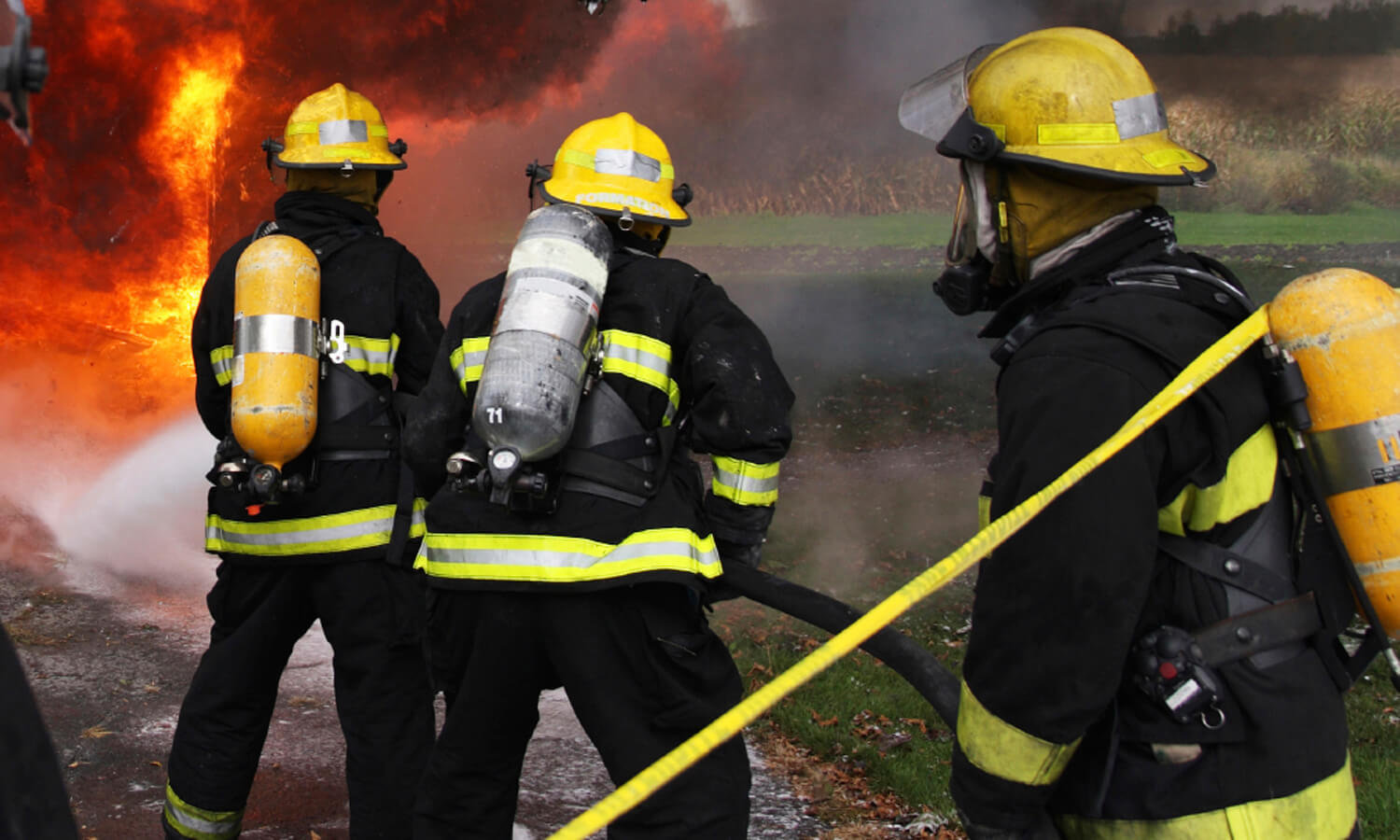
Apollo Gerolymbos, Head of Data Analytics at London Fire Brigade: “This analysis let us target inspections on a household level. It means we can be sure our fire stations are as prepared as possible for the most likely future demand on our service. Without machine learning techniques we cannot make the best use of the intelligence available to us to target risk.”
Tudor Thomas, the ASI Fellowship’s data scientist, said “The UPRN was the perfect way to link data from multiple sources. The predictive power of a machine learning model is only as good as the data we put in. To that end, Energy Performance Certificate data was important in predicting where London’s next fire might occur.”
Read more about this project at https://blog.geo.place/2018/10/25/predicting-and-preventing-londons-next-fire-using-predictive-analytics-and-the-unique-property-reference-number/.